Optimizing SwiftMR™ Protocols for Diverse Applications
Authors
Geunu Jeong1
1 – MD, Head of SwiftMR™ Research
Magnetic Resonance Imaging (MRI) : Game of Balance Between Image Quality and Scan Time
Key features of MR image quality include signal-tonoise ratio (SNR), resolution, artifact, and contrast. It is well known that these features have a trade-off relationship with scan time – meaning that compromise from at least one of these features is necessary to reduce scan time. Similarly, achieving improvement on one of the four factors without increasing scan time would also require the expense of other three parameters. This relationship was the standard on which the industry and the clinic revolved around – before SwiftMR™*.
Introducing SwiftMR™ : Enhancing MR Image Quality Without Compromise
SwiftMR™** is a deep learning-based technology which allows the user to break the conventional rule of the trade-off. SwiftMR™ is capable of enhancing the SNR and spatial resolution of MR images without extra scan time, and without sacrificing contrast or introducing artifacts. SwiftMR™ can be utilized to address various clinical needs. However, protocol setting strategies tailored to meet these specific needs must be established beforehand. The guiding principle is: “adjust the parameters to achieve desired outcomes by willingly sacrificing either SNR or spatial resolution. SwiftMR™ will compensate for any loss in SNR or spatial resolution.”
Next, different strategies for SwiftMR™-optimized protocol settings under various clinical needs will be illustrated.
Scan Time Reduction
Naturally, scan time reduction is the most common, unanimous need in modern MR imaging. Here, we will talk about strategies for a common 2D fast spin echo (FSE) or turbo spin echo (TSE) pulse sequences. The scan time of the 2D TSE pulse sequence is determined by the following formula:
where TR stands for repetition time, # TSE shots and #Phase Encoding Steps each refer to the numbers used per slice per average, # Averages indicates the number of averages and # Acquisitions denotes the number of slice sets in multi-slice imaging. From this formula, several strategies can be taken to reduce scan times.
First approach is to decrease the number of averages. This will directly reduce scan time at the expense of SNR, which SwiftMR™ is capable of recovering. While it is the simplest method to implement, factors such as worsening motion/flow artifact and free induction decay (FID) artifact should be accounted for.
Parallel Imaging (PI) is another method that reduces scan time by decreasing the number of phase encoding steps. This sacrifices SNR. While the SNR sacrifice from reducing the number of averages is √constant at scan time reduction ratio, the SNR sacrifice from using PI is equal to or more severe than reducing the number of averages and varies depending on the imaging scenario. There are primarily two conditions related to scan parameters that can minimize the SNR sacrifice (i.e., bring it closer to √scan time reduction ratio). The first is whether the phase encoding direction and the receiver coil element arrangement are parallel. For instance, when using a knee coil which surrounds the anatomy, having the phase encoding direction as Right-Left (RL) or Anterior-Posterior (AP) is much more advantageous than Head-Foot (HF). The second is whether the phase acquisition field-of-view (FOV) is sufficiently large. This refers to a phase FOV that considers phase oversampling, and the larger this value, the more advantageous it is for PI. Aside from scan parameters, another important factor is whether the number of channels available in the receiver coil is sufficient. With these conditions met (or favorable), it is possible to achieve an SNR sacrifice close to √scan time reduction ratio even with a higher PI factor, which SwiftMR™ could recover. However, using PI should also take into account the possibility of residual aliasing artifacts.
Another strategy would be to decrease the phase oversampling, which also reduces the number of phase encoding steps, thereby shortening scan time. This sacrifices SNR, which SwiftMR™ can recover. Reducing phase oversampling beyond the point where small FOV aliasing artifact occurs should be avoided.
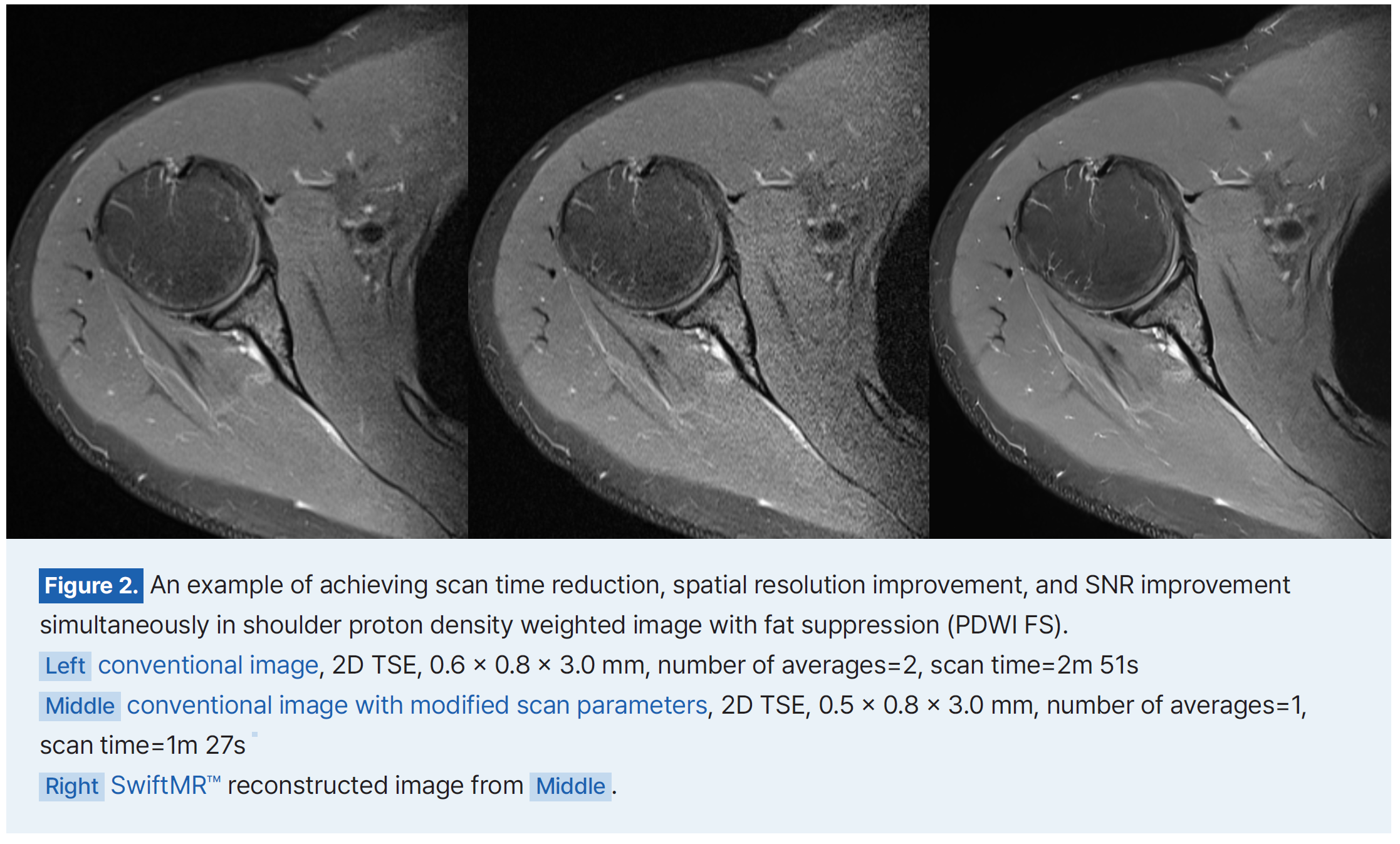
*Jeong, Geunu, et al. “All-in-One Deep Learning Framework for MR Image Reconstruction.” arXiv preprint arXiv:2405.03684 (2024)
**The intended use may vary by country.