Written by Woojin Jung
Research Scientist in AIRS Medical
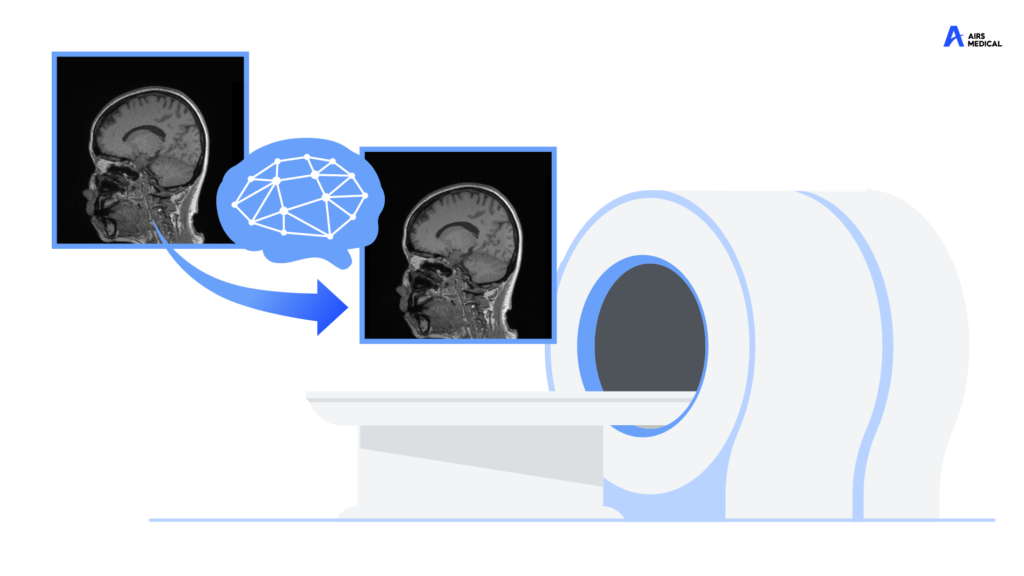
Artificial intelligence (AI) has made its debut in the clinical environment, where breakthroughs in numerous fields such as lesion detection, diagnosis, prognosis and workflow/throughput enhancement has been recently presented. AI allows reconstruction of MRI (Magnetic Resonance Imaging) images captured through accelerated imaging to a quality comparable to or even surpassing those obtained from the previous clinical standard scans. How does AI reconstruct the image quality compromised by accelerated imaging? To shed light on this principle, we’ll explore the paper titled “MR-self Noise2Noise: self-supervised deep learning–based image quality improvement of submillimeter resolution 3D MR images,” published by AIRS Medical in European Radiology.
MRI scan inherently requires a balance between scan time and image quality. Reducing the scan times can lead to poorer image quality, making diagnosis difficult. On the other hand, aiming for better image quality often results in longer scan times, which can be challenging for patients. In other words, short MRI scan times are accompanied by a degradation in image quality. Let’s take a closer look at how the quality is compromised. Would you like to see the figure below first?
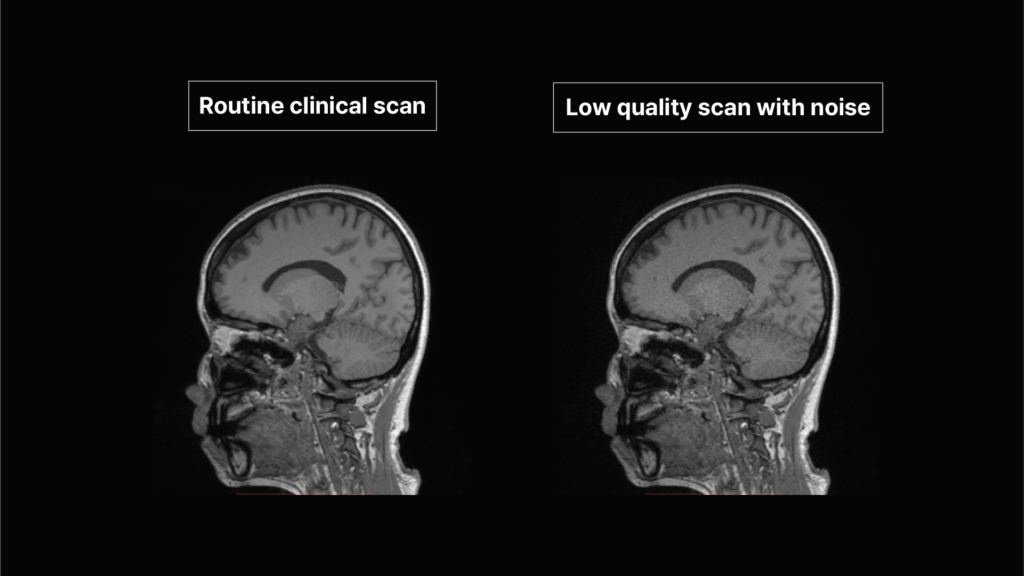
As shown in the figure, images with short MRI scan times often have a grainy appearance, which is referred to as being “noisy”. Excessive noise level often make accurate diagnosis challenging. To address this, AI techniques can be utilized to remove only the noise without degrading how the anatomical structures are depicted in the image. Then, how does AI distinguish between noise and anatomical structures?
The performance of AI primarily depends on how much data it learned from. For instance, if we have large number of MRI brain image pairs—one with heavy noise and the other without noise (clean image)—, the AI can compare these pairs to differentiate the patterns of brain structures and noise, enabling the AI to learn the process of removing noise.
However, preparing such a dataset is nearly impossible. First, due to the nature of MRI imaging techniques, MRI images inherently contain some degree of noise. Second, obtaining “clean-like” images requires significantly long scan times, which is impractical in clinical setting.
Then, can we train AI without clean images? This question has been addressed in the field of computer vision, and we were able to get inspiration from the “Noise2Noise” technique announced by NVIDIA in 2018.1 Unlike conventional methods requiring pairs of (noisy-clean) images, Noise2Noise performs equally well or even better using pairs of (noisy -noisy) images with different patterns. This means that AI can reconstruct clean images without learning from clean image data!
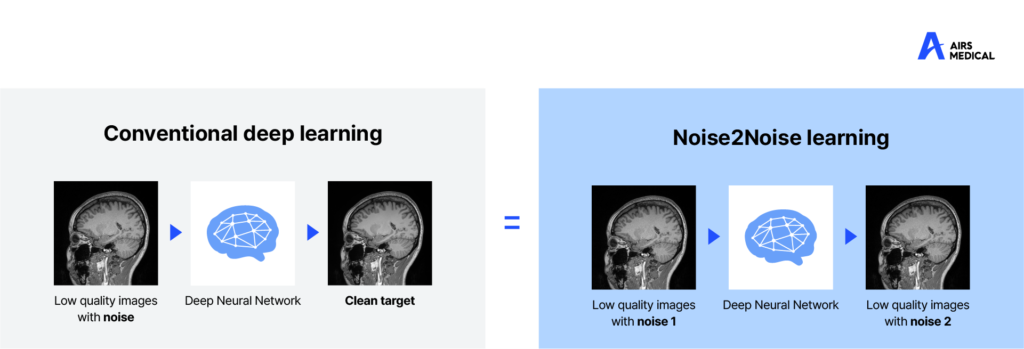
How does it work? The rationale behind this phenomenon is based on the AI’s ability to find the mean of data distribution during training. Let me provide a simple example to help you understand.
Imagine you are measuring your daily commute every morning. Sometimes it might take 30 minutes, and sometimes an hour due to traffic jam. By collecting a large amount of data, you can calculate the average commute time would be 45 minutes.
Now let’s apply this principle to the Noise2Noise technique. In this case, the average commute time corresponds to the “clean image,” while traffic conditions correspond to the “noise.” The Noise2Noise technique utilizes the fact that the average commute time (clean image) can be calculated by collecting commute time in numerous traffic situations (noise) and averaged. In other words, the average of images with different types of noise will be the same as the clean image. By training the AI with a large number of image pairs containing different types of noise, the AI learns that the average of these images corresponds to a noise-free image.
The paper published by AIRS Medical proposed an algorithm that adapted the Noise2Noise technique to MRI reconstruction.2 By applying mathematical algorithms to the raw data obtained during MRI scans, two images with different noise distributions were generated. Then, the AI model can be trained by a large number of such noisy image pairs.
When the proposed AI model was applied to the clinical practice, the results were remarkable. The structures with noise became much clearer.
To evaluate whether the AI model distorted the anatomical structures, two experienced radiologists conducted image evaluations. The conclusion was that the AI significantly improved the image quality, fine structure delineation, and lesion conspicuity without generating artifacts.
One might think that AI development is as simple as just collecting pairs of noisy and clean images for training. However, now you may have realized that a combination of complex mathematical theories and algorithms is required to build a reliable AI. If you’re interested in the more detailed explanation of the principles behind AI-based MRI image reconstruction, we recommend reading the paper we introduced today! (https://doi.org/10.1007/s00330-022-09243-y)
References:
- Lehtinen, Jaakko, et al. “Noise2Noise: Learning image restoration without clean data.” arXiv preprint arXiv:1803.04189 (2018).
- Jung, Woojin, et al. “MR-self Noise2Noise: self-supervised deep learning–based image quality improvement of submillimeter resolution 3D MR images.” European Radiology 33.4 (2023): 2686-2698.